The Importance of Data Integrity in COVID-19 Clinical Trials
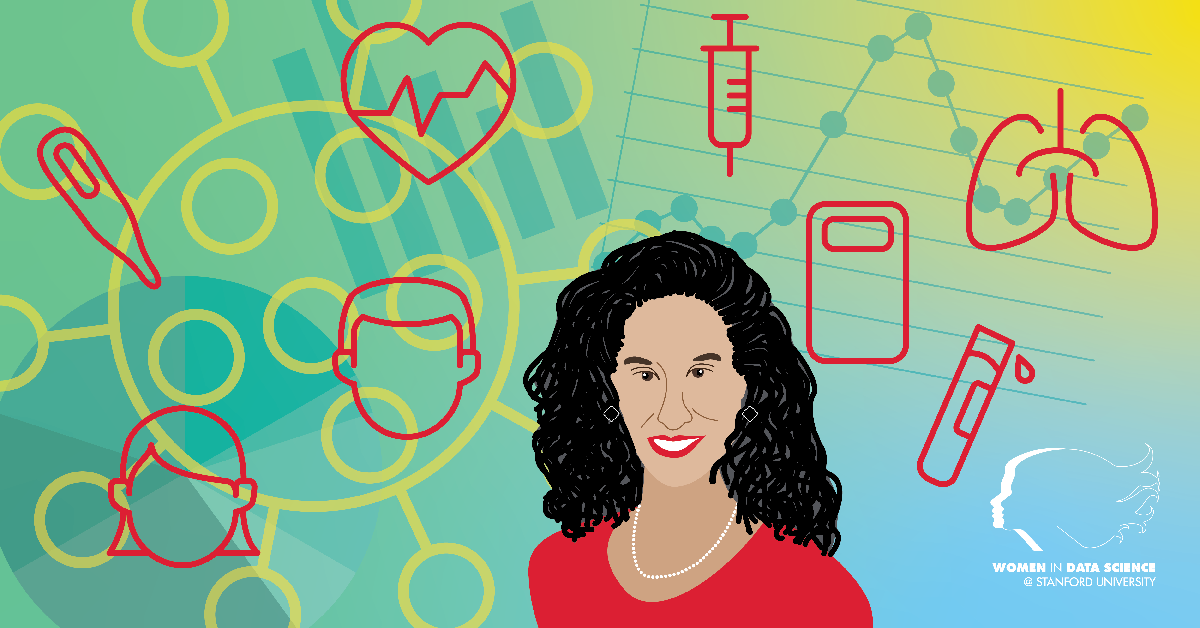
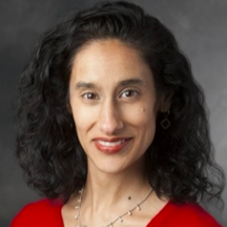
Professor of Medicine & Biomedical Data Science and Director of the Quantitative Sciences Unit at Stanford University
About this episode
Manisha Desai, professor of medicine and biomedical data science at Stanford University, shares some insights about the challenges and progress of current COVID-19 clinical trials.
Highlights
Manisha Desai is a professor of medicine (research) and of biomedical data science, and director of the Quantitative Sciences Unit at Stanford University. She is an expert in the design and analysis of clinical trials and epidemiologic studies across multiple diseases, including COVID-19. In this podcast, she provides some insights into the challenges and progress of COVID-19 clinical trials.
When COVID happened, Manisha knew her team’s expertise in clinical trials would allow them to get up to speed quickly to study this new disease. So, she augmented her team with additional data science experts to focus on trials to identify safe and effective drugs to help prevent hospitalization and disease progression. “This whole period has been so anxiety-inducing for so many of us, it’s actually been nice to focus that energy and anxiety towards work that could be part of the solution to some of the crisis,” she says. In studies so far, they have learned that there’s a real disparity in outcomes by ethnicity and race and they are trying to determine if that’s due to genetic, cultural, or environmental factors. They are also launching trials to figure out questions about immunity, seroprevalence and incidents.
Clinical trials are the gold standard for identifying safe and effective therapies. Since these trials require a lot of time and rigor, the findings have high internal validity. In a typical clinical trial, there is a sequential plan which starts by designing the study, setting up a data and safety monitoring board, database capture, and analysis plan. It’s a totally different in a pandemic when you want to launch all of these events quickly, so developing the analysis plan and designing the study don’t happen sequentially. “You’re talking to the FDA and the IRB at the same time that you’re developing the protocol, just as the ideas are sort of coming together. Everyone needs the study launched, we’re all sheltering in place and solutions need to be found, so everyone is rushing to do this,” she says.
She cautions that we need to be careful. “These protocols are in place for the safety of the patients, and the integrity of the data and the study. The worst thing would be spending all these resources, having patients contribute to the trial, and then at the end of the day having inconclusive evidence,” she says. People are anxious about finding answers, they want to publish and disseminate their findings and be the first to solve the problem. And as data scientists, it’s critical to ensure the rigor, that the study is designed well, that the limitations are understood and that there’s an appropriate interpretation of the findings. All of the misinformation that has been going on during this pandemic can contribute to further loss of lives.
Collaboration on COVID-19 research across groups is essential because we need to pool data. When you combine data across institutions and countries, we need a level of certainty about who is collecting what, how often, on whom, under which policy. This will really impact how and if you even can pool the data, and how you can interpret it. She says one of the most important foundations to the success of the research is ensuring that the data has integrity. People spend so much time spent thinking about the models but we need to get the data management process right before applying the models.
There is not currently one centralized, robust data repository in the US that makes it easy for researchers to study questions when they arise. Manisha says people are trying to get these registries up and going quickly, and it’s not easy because we ascertain data differently across different sites and countries. This is a very worthwhile investment to put time into thinking about how to collect data in standardized forms.
Manisha has seen an enormous response to solving the COVID crisis. She is optimistic that we will identify safe and effective treatments and a vaccine on the horizon. “We’ve learned that the FDA and regulatory agencies can be quite responsive. And maybe even in a non-COVID world, we can learn about how to gain efficiency and have some work arounds when necessary, and get rid of things that might be unnecessary. There will be many lessons learned, not just for COVID, but there will be other pandemics, and we will be able to learn from this experience.”
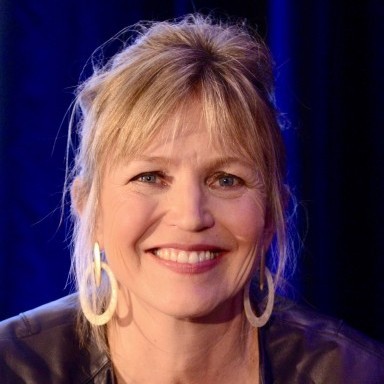
About the Host
Margot Gerritsen
Stanford Professor [Emerita] Margot Gerritsen is the Executive Director and co-founder of Women in Data Science Worldwide (WiDS) and born and raised in the Netherlands. Margot received her MSc in Applied Mathematics from Delft University of Technology before moving to the US in search of sunnier and hillier places. In. 1996 she completed her PhD in Scientific Computing & Computational Mathematics at Stanford University and moved further West to New Zealand where she spent 5 years at the University of Auckland as a lecturer in Engineering Science. In 2001, she returned to Stanford as faculty member in Energy Resources Engineering. Margot was the Director of the Institute for Computational & Mathematical Engineering (ICME) at Stanford from 2010-2018 and the Senior Associate Dean for Educational Affairs in Stanford’s School of Earth Sciences from 2015-2020. In 2022, Margot took Emerita status to devote herself to WiDS full time. Margot is a Fellow of the Society of Industrial & Applied Mathematics, and received honorary doctorates from Uppsala University, Sweden, and the Eindhoven University of Technology in the Netherlands. She now lives in Oregon with her husband Paul.
Connect with Margot Gerritsen on Twitter (@margootjeg) and LinkedIn.
Find out more about Margot on her Stanford Profile.