Alternative approaches to A/B Experiments – 3 Causal Impact Approaches | Jennifer Vlasiu
About This Video
Make answering ‘what if’ analysis questions a whole lot easier by learning about state-of-the-art, end-to-end applied frameworks for causal inference.
We will cover:
Microsoft’s “Do Why” Package Causal Impact in Python – DoWhy | An end-to-end library for causal inference — DoWhy | An end-to-end library for causal inference documentation (microsoft.github.io)
Bayesian Causal Impact in R
MLE Causal Impact in Python
Bonus: AA Testing, when to use and why it matters
We will apply these models in the context of understanding the impact of a marketing rewards campaign, as well as understand the impact from a product/feature upgrade
This workshop was conducted by Jennifer Vlasiu, Data Science & Big Data Instructor at York University
Useful resources for this workshop:
– https://bit.ly/github_casual_impact
In This Video
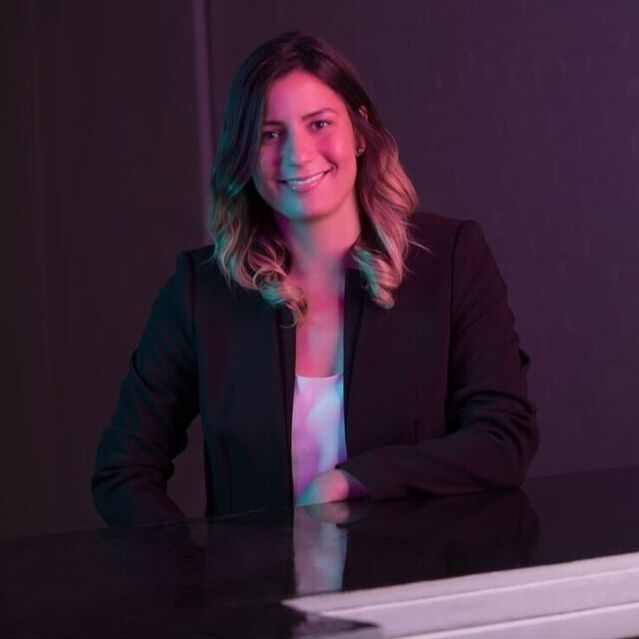
Data Science & Big Data Instructor, York University
Jennifer is a Data Scientist & Advance Analytics Strategy professional, currently focused on decision intelligence, quasi-experimentation, and model/feature/cloud solution deployments. Jennifer has over 10 years of experience in a data science, analytics and operations capacity. Her passion for business, and emerging technologies has been refined over the years via strategic and data science roles at leading global organizations. Her past engagements include Bell, ABInBev, Uber, PepsiCo, JP Morgan and the U.S. Department of Commerce.
Jennifer is passionate about digital literacy, serving as a Chapter Lead for a national non-profit, Canada Learning Code, and as a course developer and instructor for numerous colleges and universities.
When not immersed in an IDE, or exploring the latest python package, Jennifer can be found sailing or going for a run along a nearby waterfront. She can be reached at jennvlasiu.com