The Multifaceted World of Data Storage
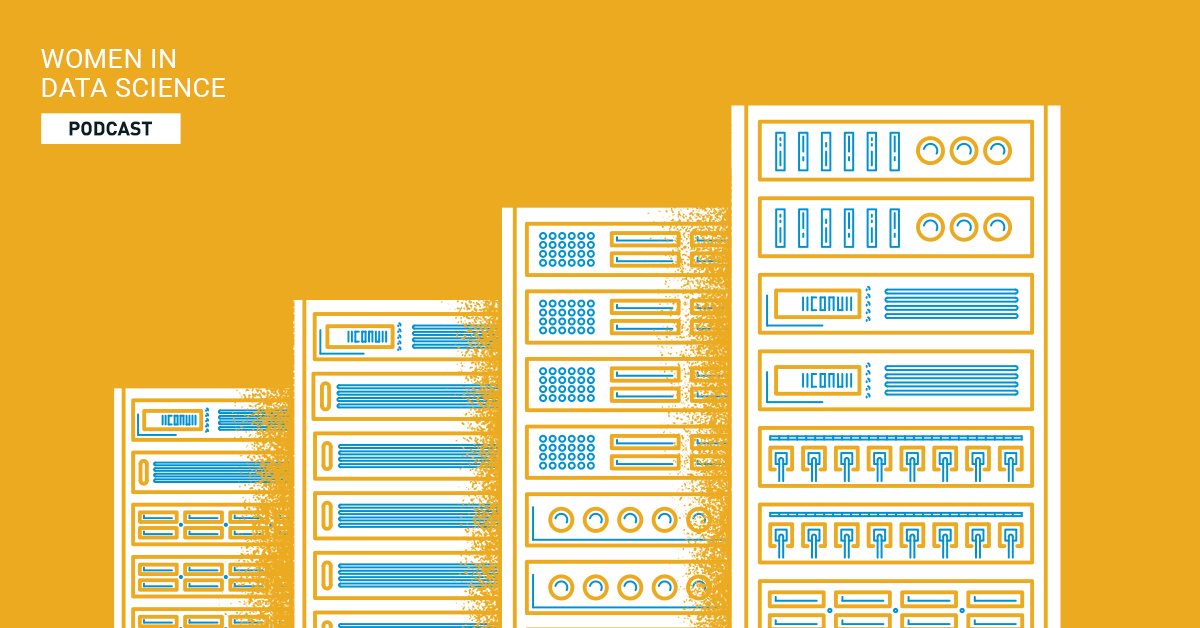
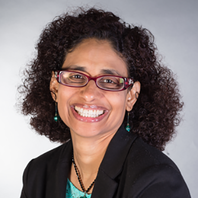
Chief Data Officer at Western Digital
About this episode
Janet George spoke to us when she was Western Digital’s chief data officer and first female Fellow. In this episode Janet explains that from manufacturing to product development, data science plays an important role in the storage industry. Janet is now the Group Vice President, Autonomous Enterprise, Advanced Analytics, Machine Learning & Artificial Intelligence at Oracle.
Highlights
“Fail fast” has become something of a mantra in Silicon Valley. But Janet George, the chief data officer of data storage giant Western Digital, has an amendment to that conventional wisdom: “Fail privately.” She suggests that failing privately allows you to open yourself up to discovery and exploration in a safe setting where you are able to take risks. “Carve out time for yourself so you can fail privately. So, you take 20 percent of your time in big initiatives you feel you can really contribute to, but take 20 percent of your time (where you can) fail privately.”
George, who has worked for some of the most important companies in the technology industry, shared this piece of advice, her career trajectory and the role of data science in the storage industry for the Women in Data Science podcast at Stanford University. Although the fear of failure is natural, it should never become a reason to avoid risk, she says. Taking an executive role at a storage company was a risk for George because she knew little about manufacturing before and. “I had to learn deeply about the device physics domain,” she says. She became familiar with arcane matters like bit counts, failure rates, temperature testing and the impact of voltage on storage cells in order to ensure her success.
Janet continued to notice how much data science came into play across the spectrum of the company’s business. From manufacturing to security and product development, “every aspect of mathematics, especially linear algebra, plays a very significant role,” she says. “When you think about the computations of scale, when you think about genetic algorithms, its applications, regression-type algorithms, or you even think about neural networks, it’s computationally heavy, it’s mathematically heavy.” Creating a die, essentially a mold, for a new storage device, for example, starts with tens of thousands of possible parameters. Data scientists at the company have to sift through a multitude of mathematical possibilities and discover the 20 or 25 most critical parameters. As the only woman at most executive meetings, she is wielding influence as a lone voice at the table, a skill honed over many years with important risks taken along the way. Her advice for aspiring data scientists: Build relationships and credibility within your organization and lead by example.