Language Models Make Stuff Up. What Should We Do About It?
About This Video
In this Tech Vision Talk, Chelsea Finn discusses when machine learning models are deployed into the world, they inevitably encounter scenarios that differ from their training data. Unfortunately, in such situations, deep neural network models, such as language models, make up answers, making the models unreliable. Even if a model makes useful predictions for many examples, such unreliability poses considerable risks when these models are interacting with real people and ultimately precludes models from being useful in safety-critical applications.
Chelsea offers a few ways that we might cope with and address the unreliability of neural network models, including a technique for detecting whether a piece of text was generated by a model and an approach for allowing models to better estimate what they don’t know so that they can refrain from making predictions when appropriate.
In This Video
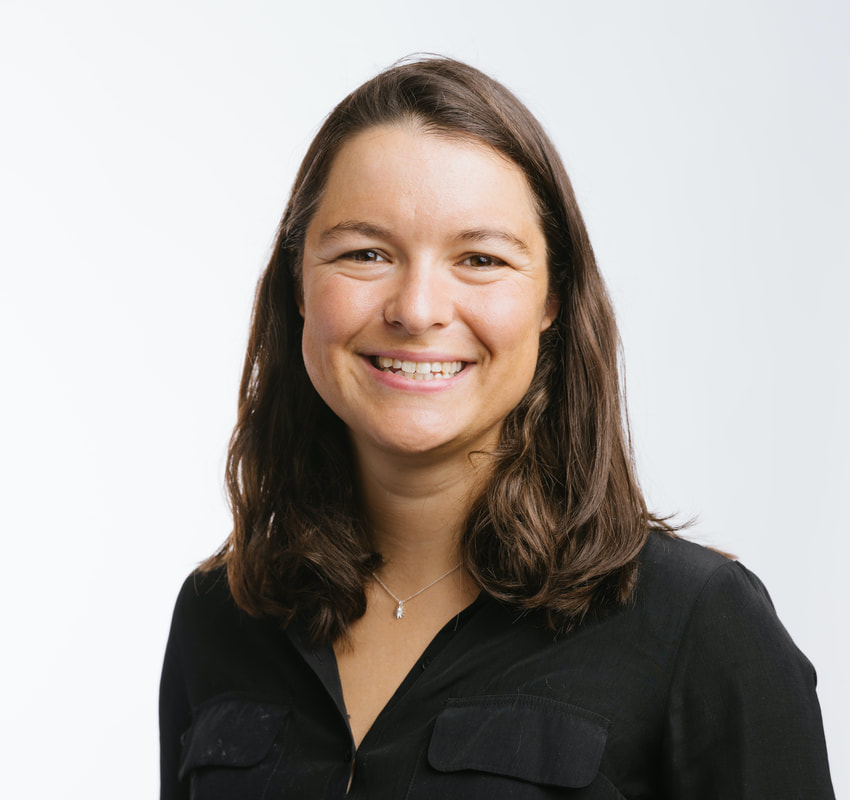
Assistant Professor in Computer Science and Electrical Engineering, Stanford University
Chelsea Finn is an Assistant Professor in Computer Science and Electrical Engineering at Stanford University, and the William George and Ida Mary Hoover Faculty Fellow. Her research interests lie in the capability of robots and other agents to develop broadly intelligent behavior through learning and interaction.