Machine Learning for Scientific R&D: Why it’s Hard and Why it’s Fun | Julia Ling
About This Video
Julia Ling, CTO at Citrine Informatics hosts a workshop on ‘Machine Learning for Scientific R&D: Why it’s Hard and Why it’s Fun’ in which she covers some of the key challenges in machine learning for R&D applications: the small, often-messy, sample-biased datasets; the exploratory nature of scientific discovery; and the curious, hands-on approach of scientific users. Julia discusses potential solutions to these challenges, including transfer learning, integration of scientific domain knowledge, uncertainty quantification, and machine learning model interpretability.
In This Video
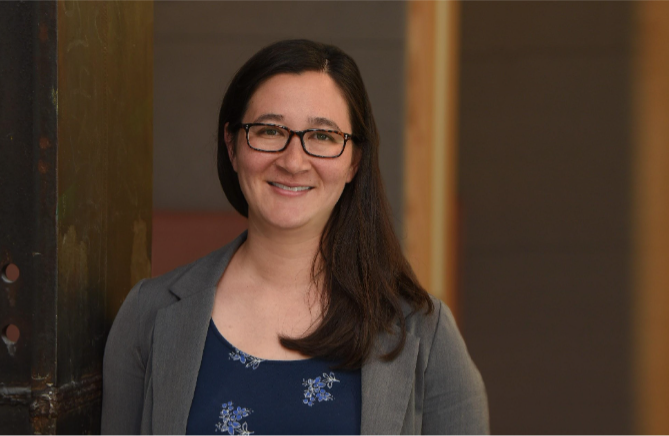
Technical Lead, Tidal
Dr. Julia Ling is the Technical Lead at Tidal, an X project focused on protecting the ocean while feeding humanity. She’s a recognized leader in applying machine learning to scientific applications. She was a Truman Fellow at Sandia National Labs where she helped pioneer the application of deep learning to turbulence modeling. Prior to Tidal, Julia was at Citrine Informatics where she led core technology development for applying machine learning to materials and chemicals development. She holds a PhD in Mechanical Engineering from Stanford University and a Bachelors in Physics from Princeton University.